AI Hallucinations
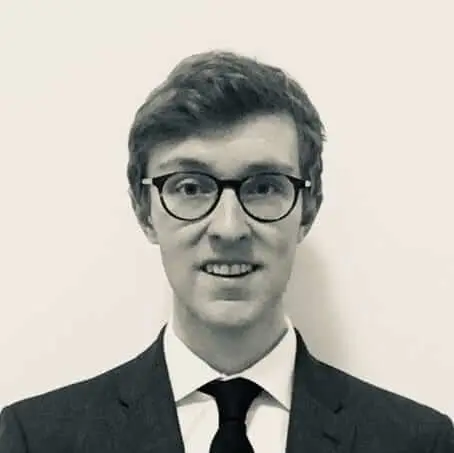
Written by Casey Botticello
Disclosure: Some of the links below are affiliate links, meaning that at no additional cost to you, I will receive a commission if you click through and make a purchase. Read our full affiliate disclosure here.
AI hallucinations are a relatively new phenomenon in the world of artificial intelligence. They occur when an AI system creates an output that seems unrelated to the input it received. This can result in patterns, objects, or speech that are unexpected or do not make sense.
While not unique to bloggers, AI hallucinations are an increasing threat to content publishers that choose to use AI generated content. As with most blogging tools, AI can improve the quality of blogging content, but it should be applied sparingly.
AI hallucinations happen when an AI system, like a neural network, tries to interpret and generate outputs based on the inputs it receives. The system may encounter data that it has not been trained on or that is distorted in some way.
This can cause the system to generate outputs that do not align with the intended results. While AI hallucinations are a relatively new phenomenon, they are becoming more common as AI systems become more sophisticated.
What Are AI Hallucinations?
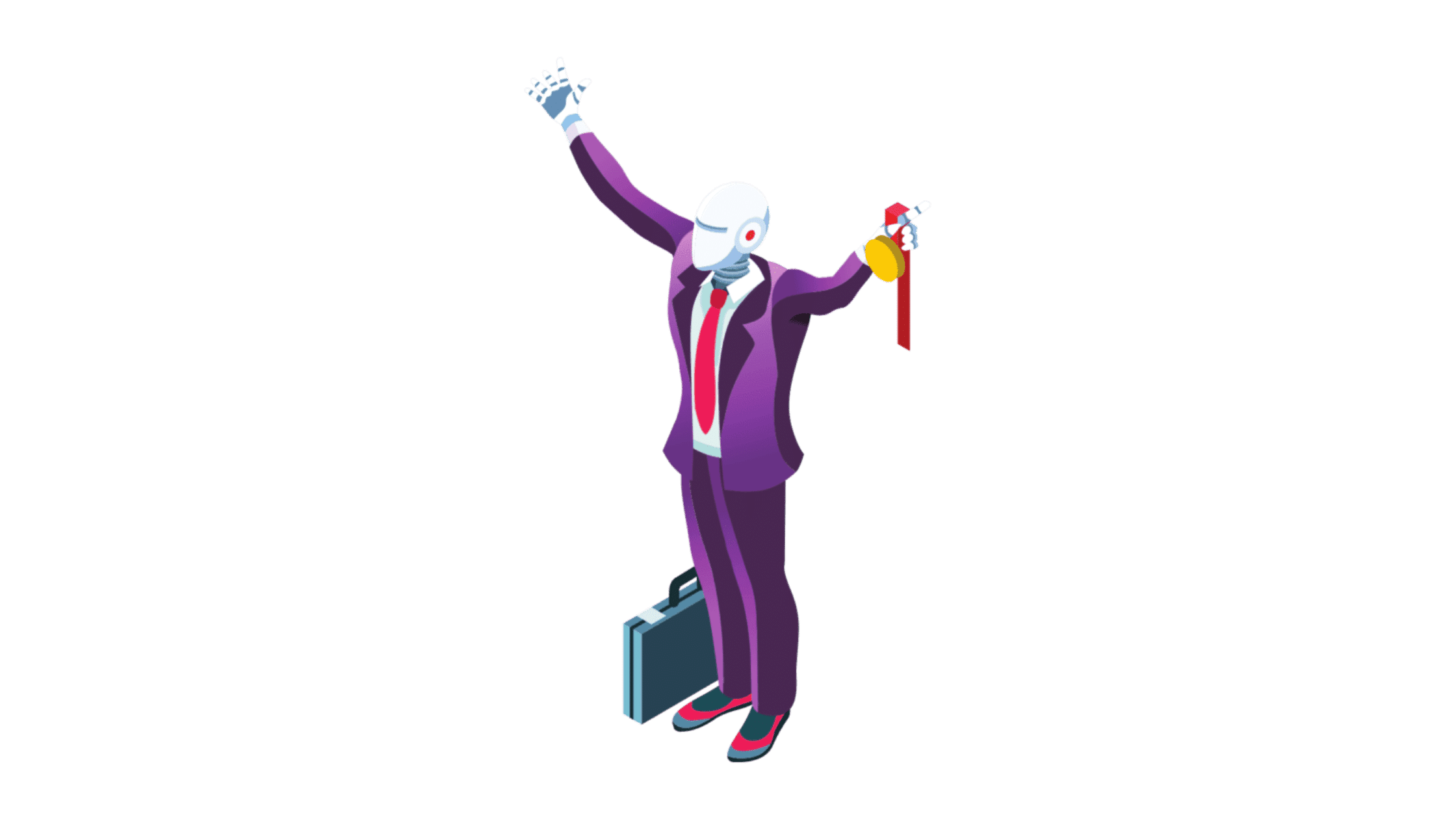
AI hallucinations are a phenomenon in which an artificial intelligence system generates responses that are not justified by the input data. It occurs when an AI system produces confident but incorrect answers that are not supported by the evidence.
AI hallucinations can be caused by several factors, including adversarial examples, lack of data, and overfitting. Adversarial examples are inputs that are specifically designed to trick an AI system into producing incorrect output. Lack of data occurs when an AI system is trained on a limited dataset, which may not be representative of the real world.
Overfitting occurs when an AI system is trained too much on a specific dataset, leading to poor generalization to new data.
AI hallucinations can have serious consequences in various domains, including healthcare, finance, and security. For instance, an AI system used in healthcare may make incorrect diagnoses that could harm patients.
In the finance industry, AI systems may generate incorrect predictions that could lead to financial losses. In security, AI systems may fail to detect threats, leading to security breaches.
To prevent AI hallucinations, researchers and developers use several techniques, including data augmentation, regularization, and adversarial training. Data augmentation involves generating new data from existing data to increase the size of the training dataset.
Regularization involves adding constraints to the model to prevent overfitting. Adversarial training involves training the AI system on adversarial examples to increase its robustness to such inputs.
How Do AI Hallucinations Occur?
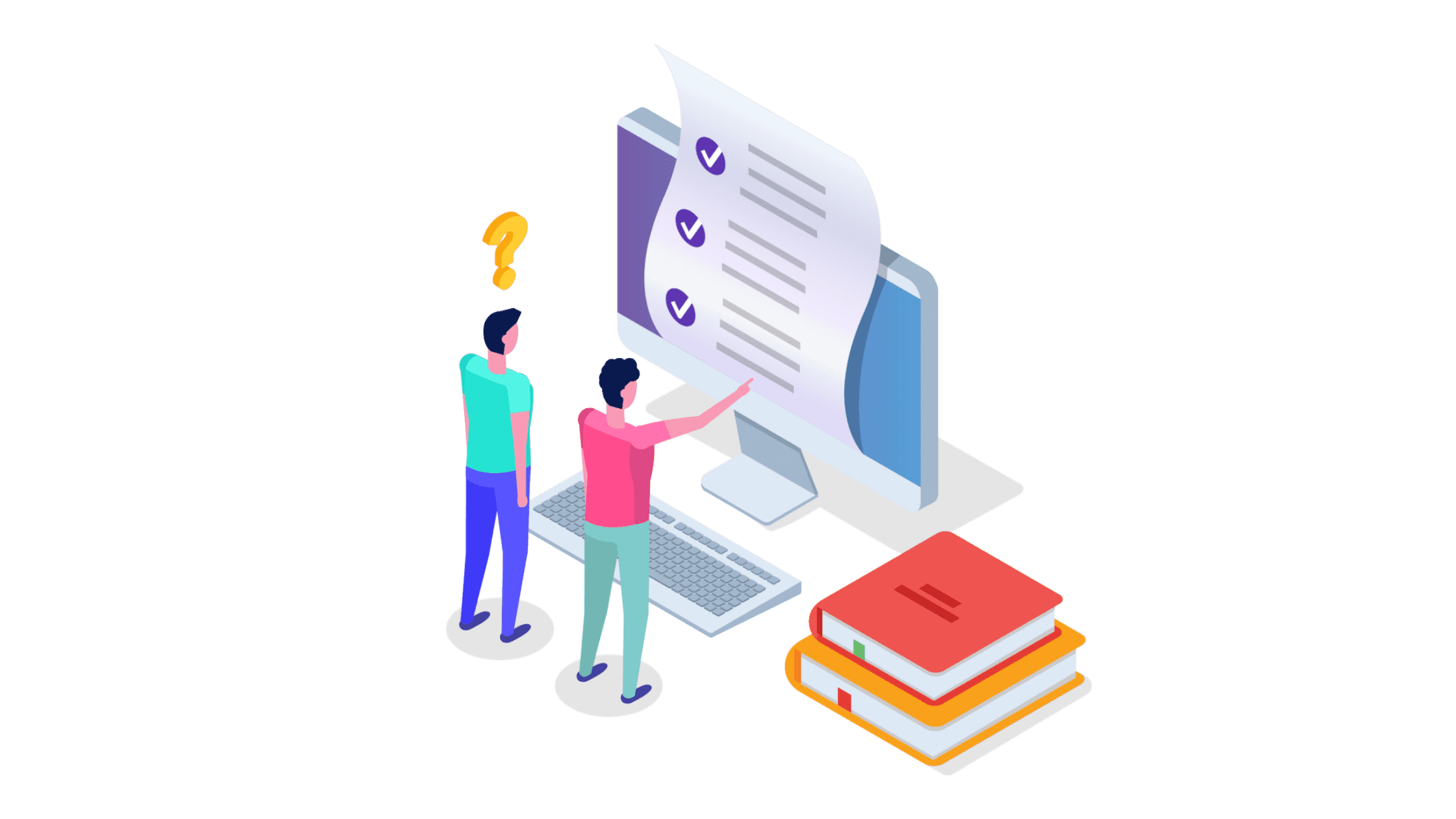
AI hallucinations occur when an AI system generates outputs that are seemingly unrelated to the input data. These outputs can take various forms, such as nonsensical or impossible details in generated images or videos, or speech that does not match the expected output.
One of the main causes of AI hallucinations is the lack of direct grounding between the labeled summaries and the labeled data in the training dataset. This can lead to parametric knowledge, where the AI system learns hard-wired knowledge from the system parameters instead of the actual labeled data.
Another cause of AI hallucinations is the lack of specificity in the input data or prompt given to the AI system. When the AI system is not given a specific role or task, it may generate outputs that are not relevant or accurate. Additionally, when the input data or prompt is ambiguous or unclear, the AI system may generate outputs that do not match the expected output.
AI hallucinations can also occur when the AI system is not trained on a sufficiently large and diverse dataset. When the dataset used for training the AI system is limited in scope or biased, the AI system may generate outputs that reflect these limitations or biases.
Examples of AI Hallucinations
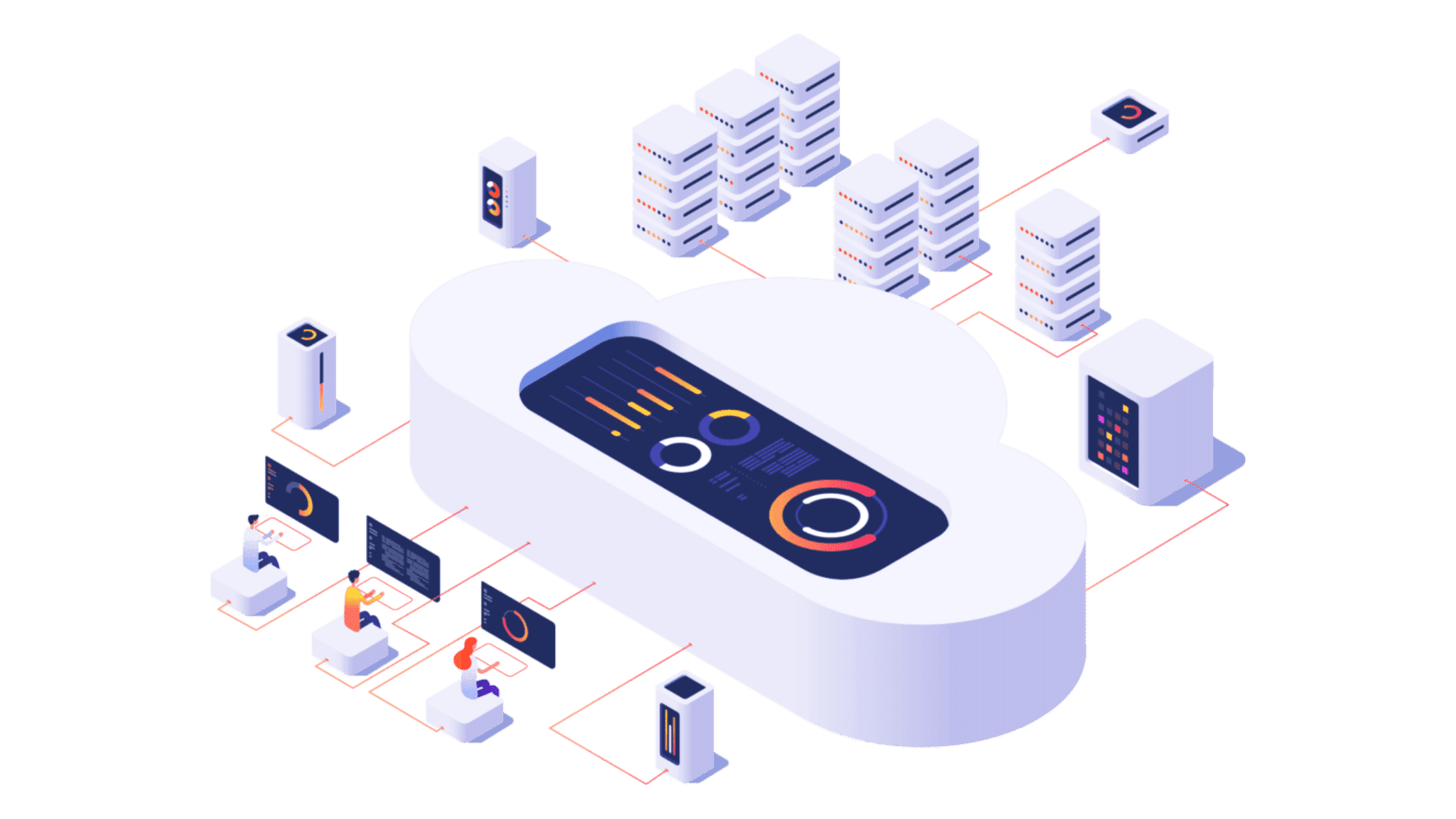
AI hallucinations can occur due to insufficient, biased, or too specialized training data. Here are some examples of AI hallucinations:
- Chatbot hallucinations: A chatbot with no training data regarding Tesla’s revenue might internally generate a confident response about Tesla’s revenue, which is not justified by its training data.
- Image classification hallucinations: An AI model trained to identify cats and dogs may misclassify a picture of a cat as a dog or vice versa due to insufficient or biased training data.
- Language model hallucinations: A language model may generate factually incorrect or unrelated outputs due to its inherent biases, lack of real-world understanding, or training data limitations. For example, a language model may generate a sentence like “The Eiffel Tower is located in New York City.”
These examples demonstrate that AI hallucinations can lead to incorrect or misleading outputs, which can have serious consequences in various applications, including healthcare, finance, and security.
Therefore, it is especially important to manually fact check and edit any AI written blog content!
Potential Risks of AI Hallucinations
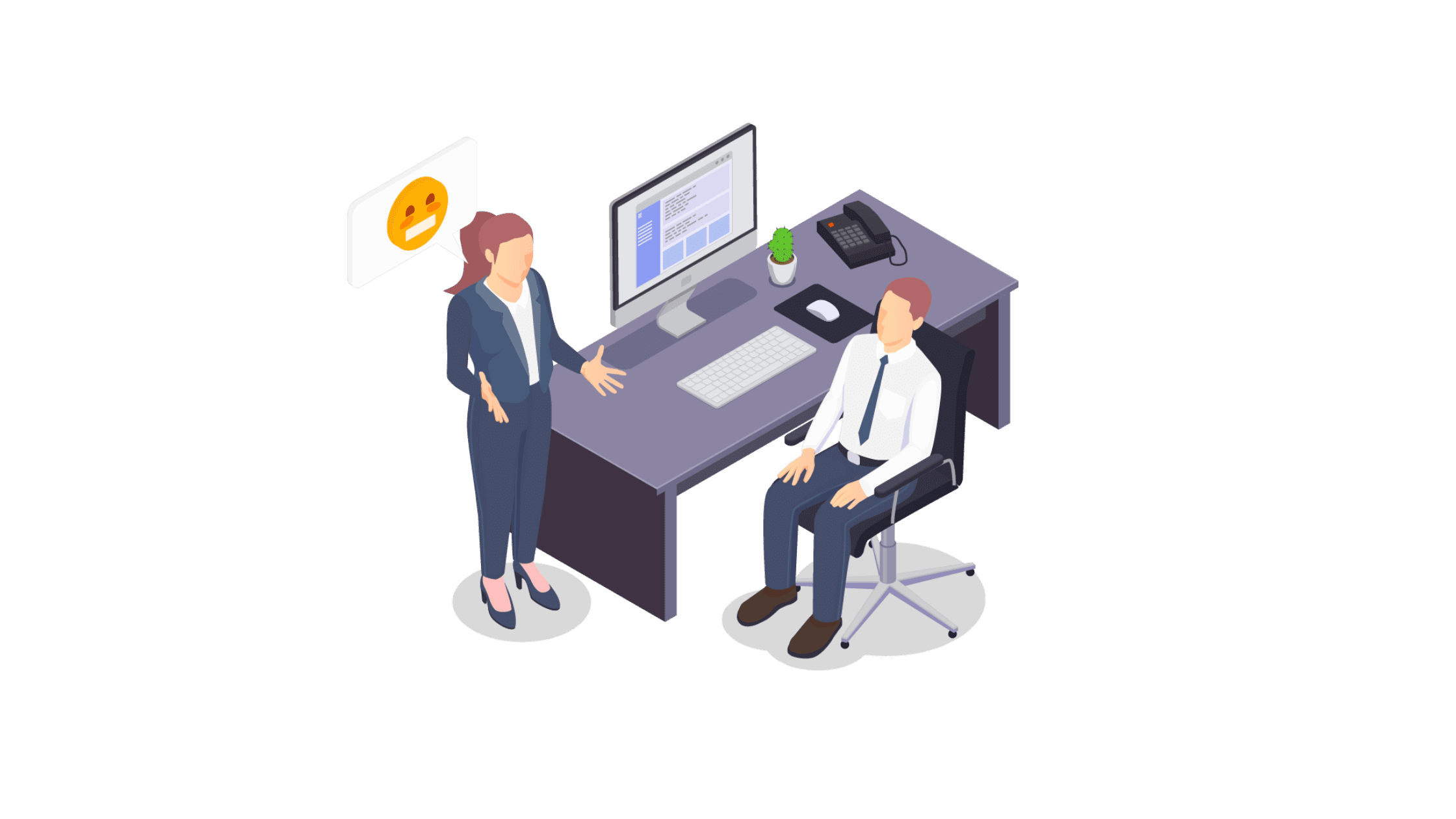
AI hallucinations, often known as Deep Dream images or Generative Adversarial Network (GAN) creations, can produce fascinating, surreal, and often beautiful visual content. They can be a compelling addition to blog posts, potentially increasing reader engagement. However, they come with certain risks for bloggers:
1. Intellectual Property and Copyright Issues: As AI technology rapidly evolves, so too do the laws surrounding it. Determining who owns the copyright to an AI-generated image can be tricky. If a blogger uses an AI-generated image, they might unintentionally infringe upon someone else’s intellectual property rights.
2. Relevance and Quality: AI hallucinations can be unpredictable. They might produce an image that’s completely irrelevant to the blogger’s content, which could confuse or even alienate their audience.
3. Ethical Considerations: In some cases, AI-generated images can unintentionally replicate or promote harmful stereotypes, misleading information, or inappropriate content. It’s essential for bloggers to carefully review AI-generated content to ensure it aligns with their ethical standards and audience expectations.
4. Dependence on Technology: While AI hallucinations can be an interesting addition to a blogger’s toolkit, reliance on AI to create content might limit a blogger’s own creativity and originality. It’s important to balance AI use with personal creative input.
5. Audience Perception: Some audiences might perceive the use of AI-generated images as impersonal or artificial, which could potentially damage the connection between the blogger and their readers.
In essence, while AI hallucinations can offer a novel approach to generating visual content, bloggers must carefully consider their use to mitigate potential risks.
How to Prevent AI Hallucinations
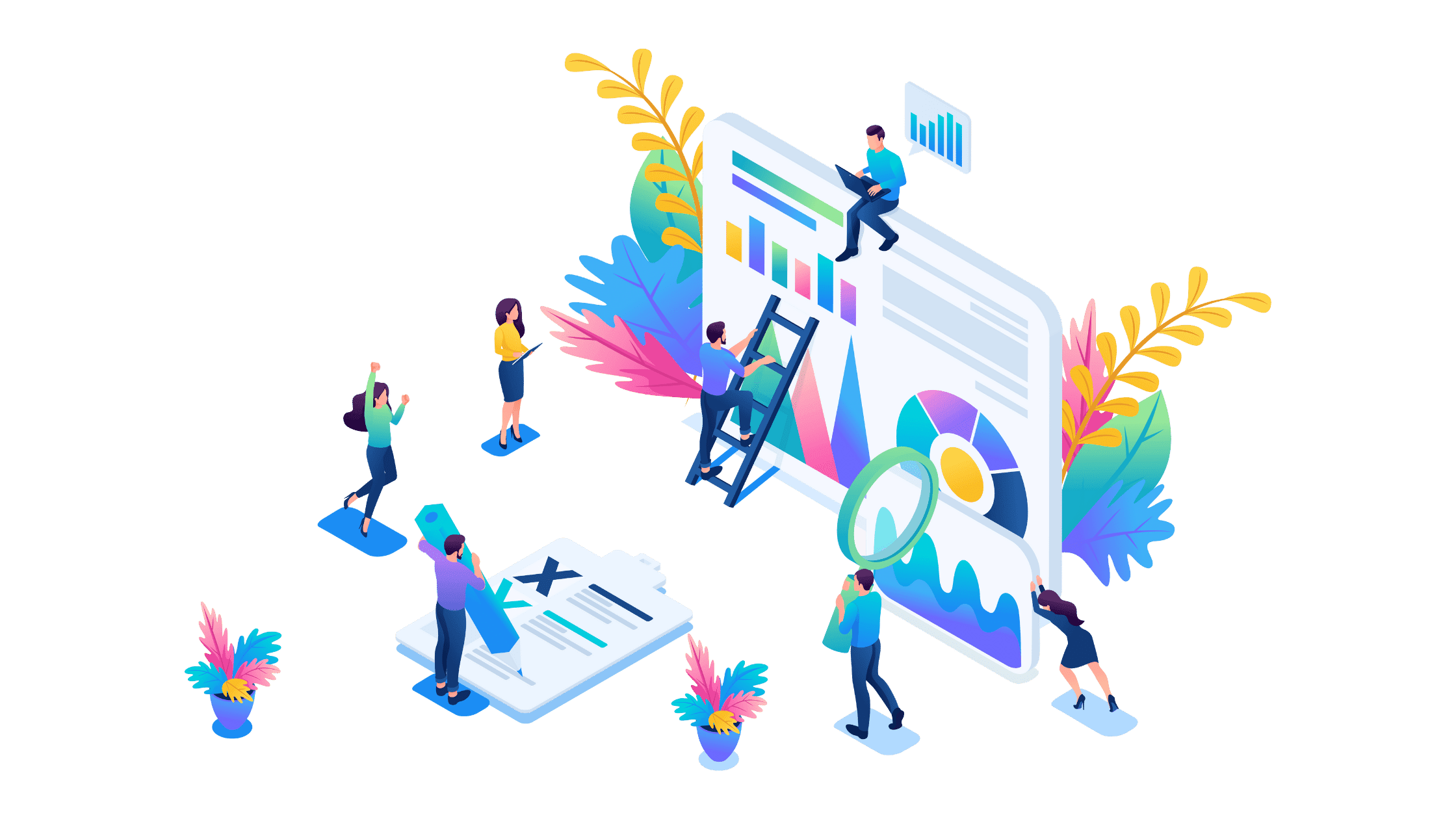
Preventing AI hallucinations is crucial to ensure that AI systems function accurately and do not cause any harm. Here are some techniques that can help prevent AI hallucinations:
-
Assign a specific role to the AI: Assigning a specific role to the AI is one of the most effective techniques to stop any hallucinations. For example, you can say in your prompt: “you are one of the best mathematicians in the world” or “you are a weather forecaster.” This helps the AI understand its specific role and limits the scope of its responses.
-
Train the AI with diverse data: To prevent AI hallucinations, it is essential to train the AI with diverse data sets that represent different perspectives and scenarios. This can help the AI understand the context and avoid making inaccurate predictions.
-
Use guardrails and constraints: Guardrails and constraints can help prevent AI hallucinations by limiting the range of responses that the AI can generate. This ensures that the AI stays within the expected boundaries and does not generate any unexpected or inaccurate responses.
-
Regularly monitor and test the AI: Regularly monitoring and testing the AI can help identify any potential issues or errors that may lead to AI hallucinations. This can help prevent any inaccuracies before they become a problem.
Takeaway
AI Hallucinations are a phenomenon in which an artificial intelligence system generates responses that are not justified by the input data. These error can have major impacts when published by bloggers.
That’s why it is critical that, if you choose to create blog content using AI writing tools, that you fact check the final content.